AI Product Management
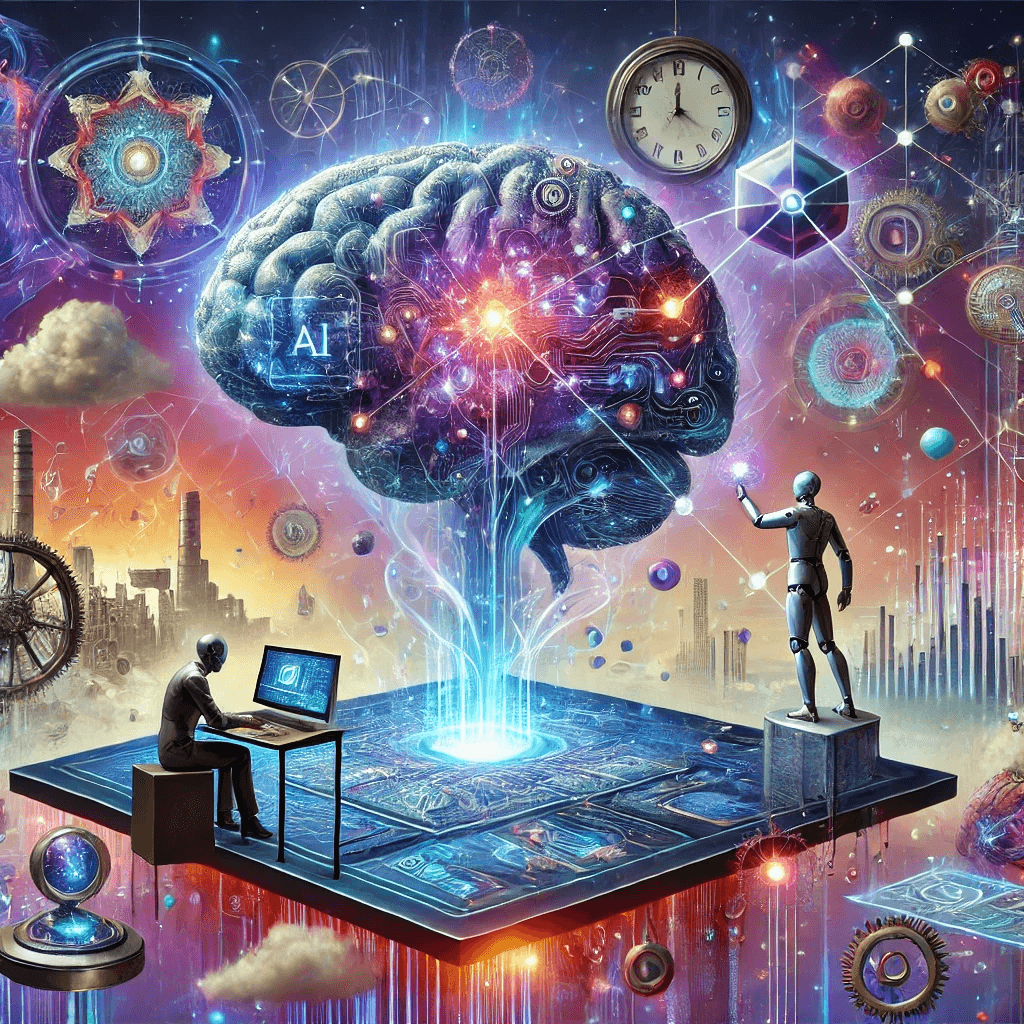
AI Product Management is a specialized subset of traditional product management that focuses on artificial intelligence technologies’ unique challenges and opportunities. Like traditional tech product management, AI product management revolves around defining, developing, and delivering products that meet user needs. However, integrating AI into the product development lifecycle introduces a set of unique dynamics that can make it more complex and rewarding.
Similarities with Traditional Product Management
At its core, AI product management shares many similarities with traditional product management. In both fields, the product manager (PM) is responsible for understanding user needs, collaborating with cross-functional teams, defining a roadmap, and ensuring the product is delivered on time and within budget. Both roles require strong communication skills, a deep understanding of customer pain points, and the ability to prioritize features and tasks based on business value.
Moreover, in both AI and traditional tech product management, the PM plays a pivotal role in bridging the gap between the technical and business sides of the company. This involves working closely with engineering, design, marketing, and sales teams to ensure the product aligns with the company’s strategic goals. Key performance indicators (KPIs) such as user engagement, customer satisfaction, and revenue growth remain essential in both contexts.
Differences in AI Product Management
While the core skills overlap, AI product management diverges significantly from traditional product management due to the unique nature of AI technology. One of the most significant differences is AI development’s iterative and experimental nature. AI models require a continuous feedback loop to improve, and success often comes from refining algorithms over time. Unlike traditional software products, where product features are usually well-defined at the outset, AI products may require ongoing model training and adjustment based on real-world data.
Additionally, AI product managers must work closely with data scientists and machine learning engineers to understand the limitations and capabilities of AI models. AI products often involve large datasets, complex algorithms, and ethical considerations that traditional products do not. For example, an AI product manager must understand the importance of data quality, model biases, and fairness to ensure that the AI operates as intended and meets ethical standards.
Keys to Success in AI Product Management
A PM needs a unique blend of technical knowledge and business acumen to succeed in AI product management. Understanding AI concepts—such as supervised vs. unsupervised learning, model training, and data preprocessing—is critical for making informed decisions about product development. While deep technical expertise may not be necessary, a solid grasp of AI fundamentals will help PMs communicate effectively with their engineering teams and make informed trade-offs when faced with technical challenges.
Furthermore, AI product managers should cultivate a mindset of continuous learning. Given the rapid pace of innovation in the AI field, staying up to date with the latest advancements, tools, and methodologies is crucial. Collaboration with data scientists and engineers is also essential. An AI PM must understand that successful AI products often require a team effort across various disciplines, and fostering a culture of collaboration is vital to overcoming the unique challenges posed by AI.
Finally, being successful in AI product management requires anticipating and mitigating risks. This includes addressing potential biases in AI models, managing data privacy issues, and ensuring the product adheres to ethical standards. It’s also essential to continuously monitor the AI’s performance post-launch, adjusting as new data comes in to ensure the product continues evolving.
AI product management is a challenging and dynamic field that requires traditional product management skills and specialized knowledge of AI technologies. By balancing technical expertise, business understanding, and a commitment to ethical considerations, AI product managers can lead the development of innovative products that transform industries.
I: Foundations of AI Product Management
- The Unique Landscape of AI Product Management
- Distinguishing AI products from traditional software products
- Key challenges and opportunities
- The AI product lifecycle
- Core competencies for AI product managers
- AI Product Strategy and Vision
- Identifying viable AI use cases
- Building an AI product roadmap
- Balancing innovation with practical implementation
- Strategic frameworks for AI initiatives
- Understanding Your AI Ecosystem
- Internal stakeholders and organizational dynamics
- External partners and vendors
- Regulatory landscape and compliance
- Building vs. buying AI capabilities
II: Technical Foundation for Product Managers
- Essential AI/ML Concepts for Product Managers
- Key machine learning paradigms
- Data requirements and characteristics
- Model development lifecycle
- Performance metrics and evaluation
- Infrastructure and Architecture Considerations
- Computing resources and scaling
- Data pipeline architecture
- Model deployment and monitoring
- Integration with existing systems
- Data Strategy and Governance
- Data quality and preparation
- Data privacy and security
- Data rights and ownership
- Building data moats
III: Product Development and Execution
- Requirements Engineering for AI Products
- Gathering and documenting AI-specific requirements
- Managing stakeholder expectations
- Defining success metrics
- Handling uncertainty and probabilistic outcomes
- User Experience Design for AI Products
- Designing for transparency and trust
- Managing user expectations
- Handling errors and edge cases
- Progressive disclosure of AI capabilities
- Agile Development in AI Projects
- Adapting agile for AI development
- Sprint planning and estimation
- Managing technical debt
- Collaboration between data scientists and developers
IV: Risk and Quality Management
- AI Ethics and Responsible Innovation
- Ethical frameworks for AI development
- Bias detection and mitigation
- Transparency and explainability
- Responsible AI practices
- Quality Assurance for AI Products
- Testing strategies for AI systems
- Performance monitoring and degradation
- A/B testing and experimentation
- Continuous improvement processes
- Risk Management and Compliance
- Risk assessment frameworks
- Regulatory compliance
- Model governance
- Crisis management and incident response
V: Go-to-Market and Operations
- Launch Strategies for AI Products
- Beta testing and pilot programs
- Phased rollout strategies
- User adoption and training
- Communication and change management
- MLOps and Production Management
- Monitoring and maintenance
- Performance optimization
- Cost management
- Incident response and recovery
- Measuring Success and ROI
- Key performance indicators
- Business impact assessment
- Cost-benefit analysis
- Long-term value creation
VI: Advanced Topics and Future Trends
- Enterprise AI Implementation Patterns
- Case studies and success stories
- Common pitfalls and lessons learned
- Industry-specific considerations
- Best practices and anti-patterns
- Emerging Technologies and Trends in AI Product Development
- Large language models and generative AI
- Edge AI and distributed systems
- AI automation and orchestration
- Future of AI product management
- Building AI Centers of Excellence
- Organizational structure and governance
- Talent development and retention
- Knowledge management
- Innovation frameworks
AI Product Management
[display-posts category=”ai-product-management”]